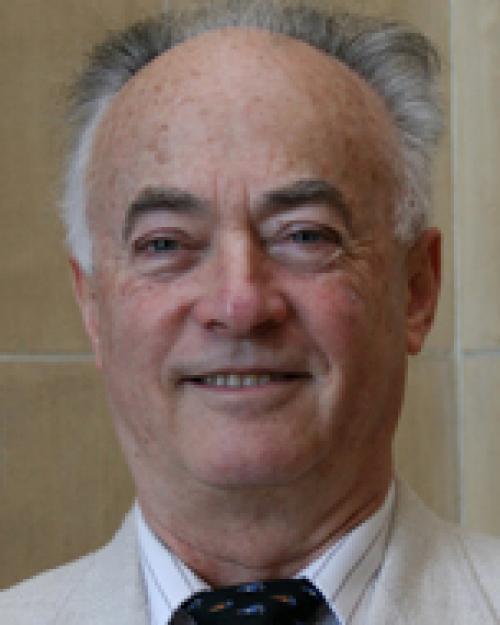
Full State Information Transfer Across Adjacent Cameras in a Network Using Gauss Helmert Filters
Presentation Menu
This lecture presents three-dimensional (3D) Cartesian tracking algorithms for a high-resolution wide field of view (FOV) camera surveillance system. This system consists of a network linking multiple narrow FOV cameras side-by-side looking at adjacent areas. In such a multi-camera system, a target usually appears in the FOV of one camera first, and then shifts to an adjacent one. The tracking algorithms estimate target 3D positions and velocities dynamically using the angular information (azimuth and elevation) provided by multiple cameras. The target state (consisting of position and velocity) is not fully observable when it is detected by the first camera only. Once it moves into the FOV of the next camera, the state can then be fully estimated. The main challenge is how to transfer the state information from the first camera to the next one when the target moves across cameras. In this paper, we develop an approach, designated as Cartesian state estimation with full maximum likelihood information transfer (fMLIT), to cope with this challenge. Since the fMLIT consists of an implicit state relationship, the conventional Kalman-like filters (for explicit constraints) are not suitable. We then develop three Gauss– Helmert filters and test them with simulation data.